Our paper titled “Hybrid physics-guided data-driven modeling for generalizable geometric accuracy prediction and improvement in two-photon lithography” has been published on Journal of Manufacturing Processes. The paper was co-authored by Sixian Jia, Jieliyue Sun, Andrew Howes, Prof. Michhelle R. Dawson, Prof. Kimani C. Toussaint Jr. , and Prof. Chenhui Shao.
Two-photon lithography (TPL) is an additive manufacturing technique to produce three-dimensional (3D) micro- and nano-scale structures. However, there exists limited research on modeling and improving the geometric accuracy of TPL. Moreover, there is a lack of understanding of the influence of TPL process parameters (e.g., scanning rate, laser power) and structure design on the resulting geometric accuracy.
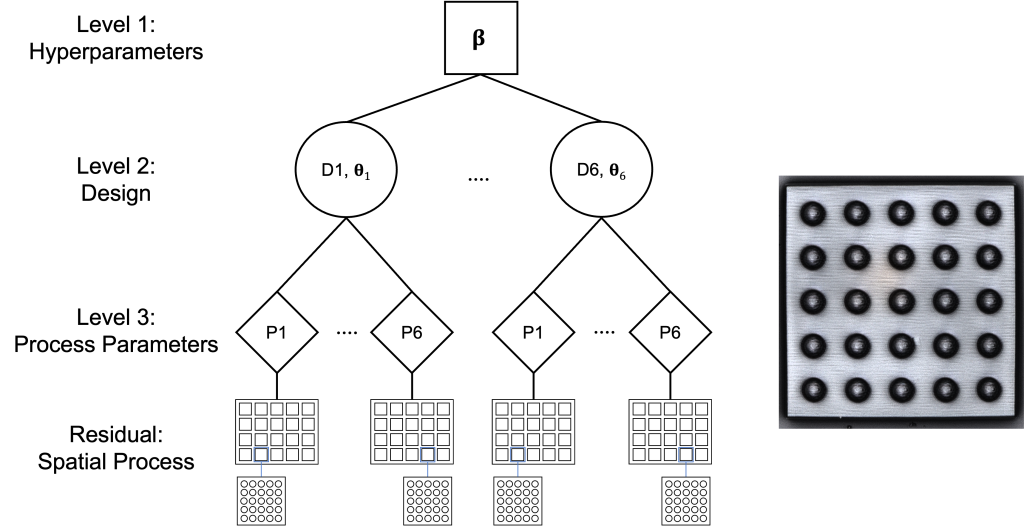
In this study, a hybrid physics-guided data-driven modeling approach is developed to predict the geometric accuracy of TPL-fabricated structures across different process parameters and different structure design dimensions. The geometric dimension is decomposed into two scales: a global trend and a spatial trend. Guided by physical laws governing voxel dimensions, the global trend is constructed as a function of TPL process parameters. Generalizable predictive modeling across structure design dimensions is enabled by the linear relationships among the model parameters. Additionally, the spatial trend term captures the spatially varying patterns in geometric features.
Read the full paper here.